The world of artificial intelligence (AI) has marked another milestone with the introduction of the Image Joint Embedding Predictive Architecture (I-JEPA), the first AI model based on the vision of Meta's Chief AI Scientist, Yann LeCun. As someone passionate about AI, I'm excited to share my thoughts on this groundbreaking model and its impact on the field, while summarizing the main points.
Revolutionizing Computer Vision
I-JEPA is a game-changer, as it learns by creating an internal model of the outside world, comparing abstract representations of images rather than pixels. This means a higher computational efficiency and strong performance across various computer vision tasks. The approach of I-JEPA can train a 632 million parameter visual transformer model with 16 A100 GPUs in under 72 hours, achieving state-of-the-art performance for low-shot classification on ImageNet with only 12 labeled examples per class.
The training cost is still high for the ordinary developers (that's about $1152 on Google Cloud preemptive). But the low shot classification result is truly a remarkable feat!
The I-JEPA model is part of a new family of Joint Embedding Predictive Architecture (JEPA) models, which aim to capture common sense background knowledge that enables intelligent behavior like sample-efficient acquisition, grounding, and planning. As AI enthusiasts, we have long been aiming for AI models to learn representations in a self-supervised way by directly using unlabeled data such as images or sounds, without relying on labeled datasets.
Pushing Boundaries in Semantics and Intuition
JEPA models like I-JEPA are pushing the boundaries in this area. Instead of using invariance-based pretraining methods, I-JEPA predicts the representation of parts of an input from other parts. By learning directly useful representations, the model can avoid biases and issues associated with generative models, which can sometimes focus on irrelevant details when trying to predict every bit of missing information.
I-JEPA predicts missing information in an abstract form, leading the model to learn more semantic features. The predictor in I-JEPA is like a primitive world model that predicts high-level information about unseen image regions, rather than pixel-level details, making it more semantically intuitive.
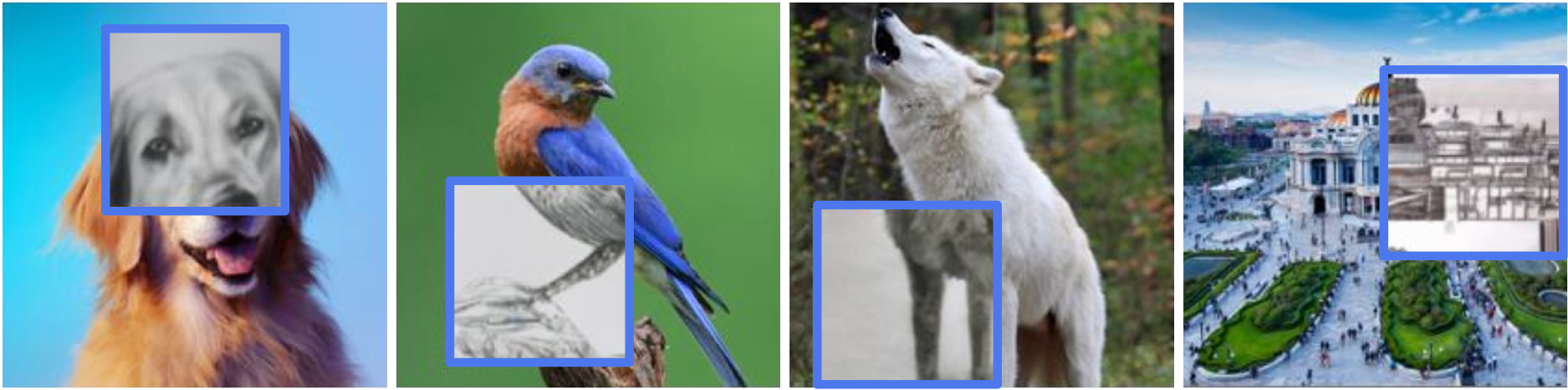
Moreover, I-JEPA pretraining is highly efficient with no overhead related to applying computationally intensive data augmentations. It learns strong off-the-shelf semantic representations and outperforms other methods on ImageNet-1K evaluations.
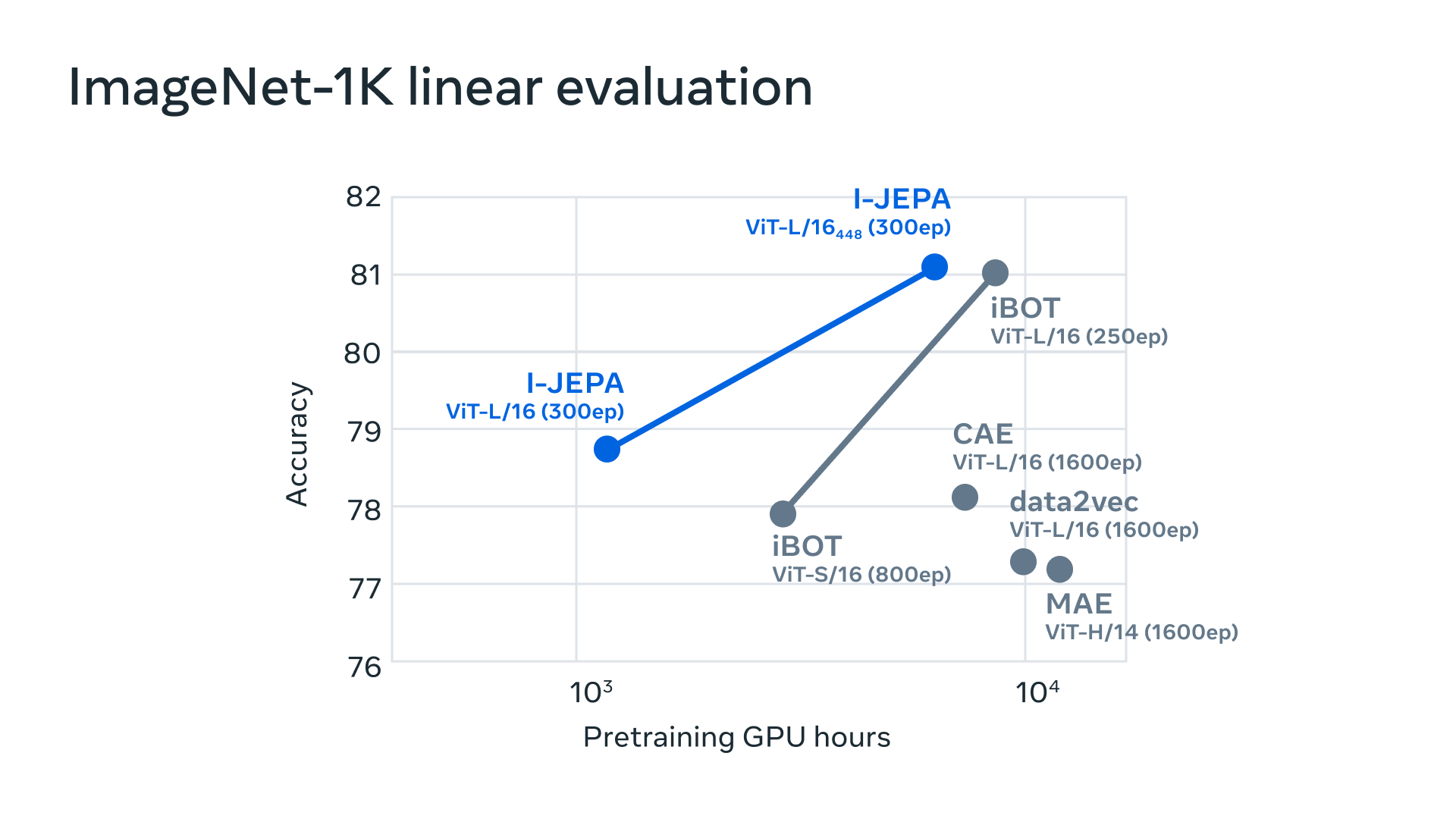
This new architecture is also versatile, with the potential to be applied to a wide range of tasks while remaining competitive with previous pretraining approaches that rely on hand-crafted data augmentations on semantic tasks.
The Future of JEPAs and Human-level Intelligence
So, what's next for JEPAs? Future advancements include learning more general world models from richer modalities, like long-range spatial and temporal predictions in videos based on short contexts and conditioning predictions on audio or textual prompts. The possibilities are endless, and I am excited to see how the JEPA approach will be extended to other domains, such as image-text paired data and video data.
As AI continues to advance, the development of groundbreaking models like I-JEPA brings us one step closer to achieving human-level intelligence in AI. With the combined efforts of brilliant minds like Yann LeCun, and the power of through JEPA models, we can expect new and imaginative uses of AI that will reshape the way we live, work, and interact with technology.
Stay tuned for more developments in the world of AI and JEPAs, as the future of artificial intelligence promises to be more exciting than ever!